Joe Ariganello VP of Product Marketing
Joe is the VP of Product Marketing at MixMode. He has led product marketing for multiple cybersecurity companies, with stops at Anomali, FireEye, Neustar and Nextel, as well as various start-ups. Originally from NY, Joe resides outside Washington DC and has a BA from Iona University.
Cybersecurity has significantly transformed by integrating Artificial Intelligence (AI) technologies. AI has revolutionized threat detection by enabling organizations to analyze vast amounts of data, identify patterns, and detect anomalies that may indicate potential cyber threats.
Early Approaches
- Expert Systems: In the early days of AI in cybersecurity, expert systems were developed to mimic human expertise and knowledge. These rule-based systems used predefined rules to identify known threats and vulnerabilities.
Machine Learning (ML) Era
- Anomaly Detection: ML algorithms gained prominence in the 2000s, enabling the development of more advanced threat detection systems. Anomaly detection techniques were employed to identify deviations from normal patterns of user behavior, network traffic, or system activities. ML algorithms could learn from historical data and adapt to new threats.
- Signature-Based Detection: ML algorithms were also used for signature-based detection, where patterns and signatures of known threats were identified and matched against incoming data. This approach effectively detected known threats but struggled with identifying unknown or zero-day attacks.
Deep Learning (DL) Advancements
- DL and Neural Networks: In the 2010s, deep learning techniques, a subset of ML, gained prominence. Deep neural networks, with their ability to process and analyze vast amounts of data, revolutionized threat detection. DL algorithms could automatically learn hierarchical representations of data, enabling more accurate detection of complex threats.
- Image and Text Analysis: DL algorithms were applied to image and text analysis, enabling the detection of malicious content, phishing attempts, and spam emails. These algorithms could analyze visual and textual elements to identify patterns and indicators of malicious intent.
Behavioral Analytics and User-Centric Approaches
- User and Entity Behavior Analytics (UEBA): AI-driven UEBA systems emerged, focusing on analyzing user behavior, access patterns, and privileges to detect insider threats, privilege abuse, and unauthorized access. These systems could identify deviations from normal behavior and flag suspicious activities.
- Contextual Analysis: AI-powered threat detection systems started incorporating contextual analysis, considering user roles, device information, and network behavior to provide more accurate threat assessments. This approach helped reduce false positives and improved the overall effectiveness of threat detection.
Integration of Threat Intelligence and Automation
- Threat Intelligence Integration: AI-driven threat detection systems began integrating with threat intelligence feeds, enabling organizations to leverage external data sources for enhanced threat detection. This integration allowed for real-time analysis of threat indicators and patterns.
- Automated Incident Response: AI-driven systems started automating incident response processes, including containment, mitigation, and recovery. By leveraging AI algorithms, organizations could respond rapidly to threats, reducing response times and minimizing the impact of cyber incidents.
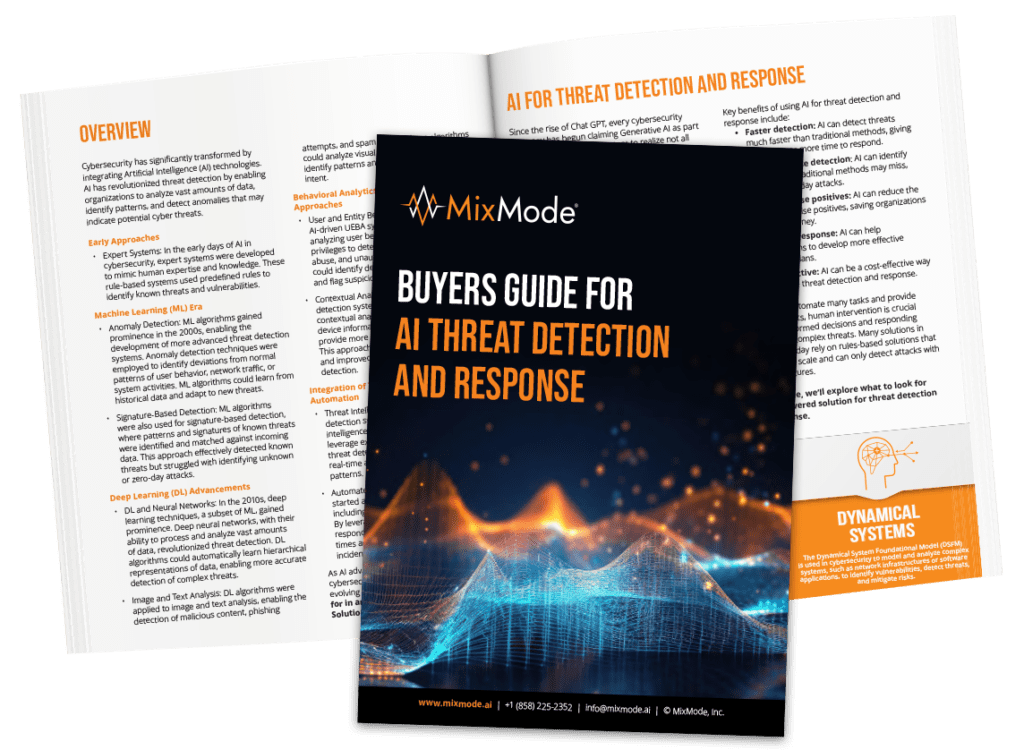
As AI advances, it will be crucial in strengthening cybersecurity defenses and staying ahead of evolving threats. So, what should you look for in an AI Threat Detection and Response Solution?
Other MixMode Articles You Might Like
Utilizing Artificial Intelligence Effectively in Cybersecurity
Bridging the Gaps: Why ITDR is the Missing Link in Identity Protection
Visibility is Not Enough to Protect Organizations from Identity Threats
Making the Most of the MITRE ATT&CK Framework: Best Practices for Security Teams
MixMode Brings Cloud-native Real-time Threat Detection and Response to the AWS Marketplace
Buyers Guide for Ai Threat Detection and Response
What to Look for When Choosing an AI Platform for Threat Detection and Response
Cybersecurity has significantly transformed by integrating Artificial Intelligence (AI) technologies. AI has revolutionized threat detection by enabling organizations to analyze vast amounts of data, identify patterns, and detect anomalies that may indicate potential cyber threats.
In this buyers guide you’ll learn about the many approaches to AI Threat detection and Response, what to look for, benefits, drawbacks, and everything you need to know when choosing an AI platform for your security operations center.
Download the Buyers Guide
Complete this form to download the eBook
Ready to join the next wave of Cybersecurity?
Stop wasting time and money with outdated threat detection solutions, get a demo of MixMode today and learn how you can improve your security capabilities.
*** This is a Security Bloggers Network syndicated blog from MixMode authored by Joe Ariganello. Read the original post at: https://mixmode.ai/blog/buyers-guide-for-ai-threat-detection-and-response/